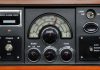
By Lance Eliot, the AI Trends Insider
There is increasing awareness about the importance of AI Ethics, consisting of being mindful of the ethical ramifications of AI systems.
AI developers are being asked to carefully design and build their AI mechanizations by ensuring that ethical considerations are at the forefront of the AI systems development process. When fielding AI, those responsible for the operational use of the AI also need to be considering crucial ethical facets of the in-production AI systems. Meanwhile, the public and those using or reliant upon AI systems are starting to clamor for heightened attention to the ethical and unethical practices and capacities of AI.
Consider a simple example. Suppose an AI application is developed to assess car loan applicants. Using Machine Learning (ML) and Deep Learning (DL), the AI system is trained on a trove of data and arrives at some means of choosing among those that it deems are loan worthy and those that are not.
The underlying Artificial Neural Network (ANN) is so computationally complex that there are no apparent means to interpret how it arrives at the decisions being rendered. Also, there is no built-in explainability capability and thus the AI is unable to articulate why it is making the choices that it is undertaking (note: there is a movement toward including XAI, explainable AI components to try and overcome this inscrutability hurdle).
Upon the AI-based loan assessment application being fielded, soon thereafter protests arose by some that assert they were turned down for their car loan due to an improper inclusion of race or gender as a key factor in rendering the negative decision.
At first, the maker of the AI application insists that they did not utilize such factors and professes complete innocence in the matter. Turns out though that a third-party audit of the AI application reveals that the ML/DL is indeed using race and gender as core characteristics in the car loan assessment process. Deep within the mathematically arcane elements of the neural network, data related to race and gender were intricately woven into the calculations, having been dug out of the initial training dataset provided when the ANN was crafted.
That is an example of how biases can be hidden within an AI system. And it also showcases that such biases can go otherwise undetected, including that the developers of the AI did not realize that the biases existed and were seemingly confident that they had not done anything to warrant such biases being included.
People affected by the AI application might not realize they are being subjected to such biases. In this example, those being adversely impacted perchance noticed and voiced their concerns, but we are apt to witness a lot of AI that no one will realize they are being subjugated to biases and therefore not able to ring the bell of dismay.
Various AI Ethics principles are being proffered by a wide range of groups and associations, hoping that those crafting AI will take seriously the need to consider embracing AI ethical considerations throughout the life cycle of designing, building, testing, and fielding AI.
AI Ethics typically consists of these key principles:
1) Inclusive growth, sustainable development, and well-being
2) Human-centered values and fairness
3) Transparency and explainability
4) Robustness, security, and safety
5) Accountability
We certainly expect humans to exhibit ethical behavior, and thus it seems fitting that we would expect ethical behavior from AI too.
Since the aspirational goal of AI is to provide machines that are the equivalent of human intelligence, being able to presumably embody the same range of cognitive capabilities that humans do, this perhaps suggests that we will only be able to achieve the vaunted goal of AI by including some form of ethics-related component or capacity.
What this means is that if humans encapsulate ethics, which they seem to do, and if AI is trying to achieve what humans are and do, the AI ought to have an infused ethics capability else it would be something less than the desired goal of achieving human intelligence.
You could claim that anyone crafting AI that does not include an ethics facility is undercutting what should be a crucial and integral aspect of any AI system worth its salt.
Of course, trying to achieve the goals of AI is one matter, meanwhile, since we are going to be mired in a world with AI, for our safety and well-being as humans we would rightfully be arguing that AI had better darned abide by ethical behavior, however that might be so achieved.
Now that we’ve covered that aspect, let’s take a moment to ponder the nature of ethics and ethical behavior.
Considering Whether Humans Always Behave Ethically
Do humans always behave ethically? I think we can all readily agree that humans do not necessarily always behave in a strictly ethical manner.
Is ethical behavior by humans able to be characterized solely by whether someone is in an ethically binary state of being, namely either purely ethical versus being wholly unethical? I would dare say that we cannot always pin down human behavior into two binary-based and mutually exclusive buckets of being ethical or being unethical. The real-world is often much grayer than that, and we at times are more likely to assess that someone is doing something ethically questionable, but it is not purely unethical, nor fully ethical.
In a sense, you could assert that human behavior ranges on a spectrum of ethics, at times being fully ethical and ranging toward the bottom of the scale as being wholly and inarguably unethical. In-between there is a lot of room for how someone ethically behaves.
If you agree that the world is not a binary ethical choice of behaviors that fit only into truly ethical versus solely unethical, you would therefore also presumably be amenable to the notion that there is a potential scale upon which we might be able to rate ethical behavior.
This scale might be from the scores of 1 to 10, or maybe 1 to 100, or whatever numbering we might wish to try and assign, maybe even including negative numbers too.
Let’s assume for the moment that we will use the positive numbers of a 1 to 10 scale for increasingly being ethical (the topmost is 10), and the scores of -1 to -10 for being unethical (the -10 is the least ethical or in other words most unethical potential rating), and zero will be the midpoint of the scale.
Please do not get hung up on the scale numbering, which can be anything else that you might like. We could even use letters of the alphabet or any kind of sliding scale. The point being made is that there is a scale, and we could devise some means to establish a suitable scale for use in these matters.
The twist is about to come, so hold onto your hat.
We could observe a human and rate their ethical behavior on particular aspects of what they do. Maybe at work, a person gets an 8 for being ethically observant, while perhaps at home they are a more devious person, and they get a -5 score.
Okay, so we can rate human behavior. Could we drive or guide human behavior by the use of the scale?
Suppose we tell someone that at work they are being observed and their target goal is to hit an ethics score of 9 for their first year with the company. Presumably, they will undertake their work activities in such a way that it helps them to achieve that score.
In that sense, yes, we can potentially guide or prod human behavior by providing targets related to ethical expectations. I told you a twist was going to arise, and now here it is. For AI, we could use an ethical rating or score to try and assess how ethically proficient the AI is.
In that manner, we might be more comfortable using that particular AI if we knew that it had a reputable ethical score. And we could also presumably seek to guide or drive the AI toward an ethical score too, similar to how this can be done with humans, and perhaps indicate that the AI should be striving towards some upper bound on the ethics scale.
Some pundits immediately recoil at this notion. They argue that AI should always be a +10 (using the scale that I’ve laid out herein). Anything less than a top ten is an abomination and the AI ought to not exist. Well, this takes us back into the earlier discussion about whether ethical behavior is in a binary state.
Are we going to hold AI to a “higher bar” than humans by insisting that AI always be “perfectly” ethical and nothing less so?
This is somewhat of a quandary due to the point that AI overall is presumably aiming to be the equivalent of human intelligence, and yet we do not hold humans to that same standard.
For some, they fervently believe that AI must be held to a higher standard than humans. We must not accept or allow any AI that cannot do so.
Others indicate that this seems to fly in the face of what is known about human behavior and begs the question of whether AI can be attained if it must do something that humans cannot attain.
Furthermore, they might argue that forcing AI to do something that humans do not undertake is now veering away from the assumed goal of arriving at the equivalent of human intelligence, which might bump us away from being able to do so as a result of this insistence about ethics.
Round and round these debates continue to go.
Those on the must-be topnotch ethical AI are often quick to point out that by allowing AI to be anything less than a top ten, you are opening Pandora’s box. For example, it could be that AI dips down into the negative numbers and sits at a -4, or worse too it digresses to become miserably and fully unethical at a dismal -10.
Anyway, this is a debate that is going to continue and not be readily resolved, so let’s move on.
If you are still of the notion that ethics exists on a scale and that AI might also be measured by such a scale, and if you also are willing to accept that behavior can be driven or guided by offering where to reside on the scale, the time is ripe to bring up tuning knobs. Ethics tuning knobs.
Here’s how that works. You come in contact with an AI system and are interacting with it. The AI presents you with an ethics tuning knob, showcasing a scale akin to our ethics scale earlier proposed. Suppose the knob is currently at a 6, but you want the AI to be acting more aligned with an 8, so you turn the knob upward to the 8. At that juncture, the AI adjusts its behavior so that ethically it is exhibiting an 8-score level of ethical compliance rather than the earlier setting of a 6.
What do you think of that?
Some would bellow out balderdash, hogwash, and just unadulterated nonsense. A preposterous idea or is it genius? You’ll find that there are experts on both sides of that coin. Perhaps it might be helpful to provide the ethics tuning knob within a contextual exemplar to highlight how it might come to play.
Here’s a handy contextual indication for you: Will AI-based true self-driving cars potentially contain an ethics tuning knob for use by riders or passengers that use self-driving vehicles?
Let’s unpack the matter and see.
For my framework about AI autonomous cars, see the link here: https://aitrends.com/ai-insider/framework-ai-self-driving-driverless-cars-big-picture/
Why this is a moonshot effort, see my explanation here: https://aitrends.com/ai-insider/self-driving-car-mother-ai-projects-moonshot/
For more about the levels as a type of Richter scale, see my discussion here: https://aitrends.com/ai-insider/richter-scale-levels-self-driving-cars/
For the argument about bifurcating the levels, see my explanation here: https://aitrends.com/ai-insider/reframing-ai-levels-for-self-driving-cars-bifurcation-of-autonomy/
Understanding The Levels Of Self-Driving Cars
As a clarification, true self-driving cars are ones that the AI drives the car entirely on its own and there isn’t any human assistance during the driving task.
These driverless vehicles are considered a Level 4 and Level 5, while a car that requires a human driver to co-share the driving effort is usually considered at a Level 2 or Level 3. The cars that co-share the driving task are described as being semi-autonomous, and typically contain a variety of automated add-on’s that are referred to as ADAS (Advanced Driver-Assistance Systems).
There is not yet a true self-driving car at Level 5, which we don’t yet even know if this will be possible to achieve, and nor how long it will take to get there.
Meanwhile, the Level 4 efforts are gradually trying to get some traction by undergoing very narrow and selective public roadway trials, though there is controversy over whether this testing should be allowed per se (we are all life-or-death guinea pigs in an experiment taking place on our highways and byways, some contend).
Since semi-autonomous cars require a human driver, the adoption of those types of cars won’t be markedly different than driving conventional vehicles, so there’s not much new per se to cover about them on this topic (though, as you’ll see in a moment, the points next made are generally applicable).
For semi-autonomous cars, it is important that the public needs to be forewarned about a disturbing aspect that’s been arising lately, namely that despite those human drivers that keep posting videos of themselves falling asleep at the wheel of a Level 2 or Level 3 car, we all need to avoid being misled into believing that the driver can take away their attention from the driving task while driving a semi-autonomous car.
You are the responsible party for the driving actions of the vehicle, regardless of how much automation might be tossed into a Level 2 or Level 3.
For why remote piloting or operating of self-driving cars is generally eschewed, see my explanation here: https://aitrends.com/ai-insider/remote-piloting-is-a-self-driving-car-crutch/
To be wary of fake news about self-driving cars, see my tips here: https://aitrends.com/ai-insider/ai-fake-news-about-self-driving-cars/
The ethical implications of AI driving systems are significant, see my indication here: http://aitrends.com/selfdrivingcars/ethically-ambiguous-self-driving-cars/
Be aware of the pitfalls of normalization of deviance when it comes to self-driving cars, here’s my call to arms: https://aitrends.com/ai-insider/normalization-of-deviance-endangers-ai-self-driving-cars/
Self-Driving Cars And Ethics Tuning Knobs
For Level 4 and Level 5 true self-driving vehicles, there won’t be a human driver involved in the driving task. All occupants will be passengers. The AI is doing the driving.
This seems rather straightforward. You might be wondering where any semblance of ethics behavior enters the picture. Here’s how. Some believe that a self-driving car should always strictly obey the speed limit.
Imagine that you have just gotten into a self-driving car in the morning and it turns out that you are possibly going to be late getting to work. Your boss is a stickler and has told you that coming in late is a surefire way to get fired.
You tell the AI via its Natural Language Processing (NLP) that the destination is your work address.
And, you ask the AI to hit the gas, push the pedal to the metal, screech those tires, and get you to work on-time.
But it is clear cut that if the AI obeys the speed limit, there is absolutely no chance of arriving at work on-time, and since the AI is only and always going to go at or less than the speed limit, your goose is fried.
Better luck at your next job.
Whoa, suppose the AI driving system had an ethics tuning knob.
Abiding strictly by the speed limit occurs when the knob is cranked up to the top numbers like say 9 and 10.
You turn the knob down to a 5 and tell the AI that you need to rush to work, even if it means going over the speed limit, which at a score of 5 it means that the AI driving system will mildly exceed the speed limit, though not in places like school zones, and only when the traffic situation seems to allow for safely going faster than the speed limit by a smidgen.
The AI self-driving car gets you to work on-time!
Later that night, when heading home, you are not in as much of a rush, so you put the knob back to the 9 or 10 that it earlier was set at.
Also, you have a child-lock on the knob, such that when your kids use the self-driving car, which they can do on their own since there isn’t a human driver needed, the knob is always set at the topmost of the scale and the children cannot alter it.
How does that seem to you?
Some self-driving car pundits find the concept of such a tuning knob to be repugnant.
They point out that everyone will “cheat” and put the knob on the lower scores that will allow the AI to do the same kind of shoddy and dangerous driving that humans do today. Whatever we might have otherwise gained by having self-driving cars, such as the hoped-for reduction in car crashes, along with the reduction in associated injuries and fatalities, will be lost due to the tuning knob capability.
Others though point out that it is ridiculous to think that people will put up with self-driving cars that are restricted drivers that never bend or break the law.
You’ll end-up with people opting to rarely use self-driving cars and will instead drive their human-driven cars. This is because they know that they can drive more fluidly and won’t be stuck inside a self-driving car that drives like some scaredy-cat.
As you might imagine, the ethical ramifications of an ethics tuning knob are immense.
In this use case, there is a kind of obviousness about the impacts of what an ethics tuning knob foretells.
Other kinds of AI systems will have their semblance of what an ethics tuning knob might portend, and though it might not be as readily apparent as the case of self-driving cars, there is potentially as much at stake in some of those other AI systems too (which, like a self-driving car, might entail life-or-death repercussions).
For why remote piloting or operating of self-driving cars is generally eschewed, see my explanation here: https://aitrends.com/ai-insider/remote-piloting-is-a-self-driving-car-crutch/
To be wary of fake news about self-driving cars, see my tips here: https://aitrends.com/ai-insider/ai-fake-news-about-self-driving-cars/
The ethical implications of AI driving systems are significant, see my indication here: http://aitrends.com/selfdrivingcars/ethically-ambiguous-self-driving-cars/
Be aware of the pitfalls of normalization of deviance when it comes to self-driving cars, here’s my call to arms: https://aitrends.com/ai-insider/normalization-of-deviance-endangers-ai-self-driving-cars/
Conclusion
If you really want to get someone going about the ethics tuning knob topic, bring up the allied matter of the Trolley Problem.
The Trolley Problem is a famous thought experiment involving having to make choices about saving lives and which path you might choose. This has been repeatedly brought up in the context of self-driving cars and garnered acrimonious attention along with rather diametrically opposing views on whether it is relevant or not.
In any case, the big overarching questions are will we expect AI to have an ethics tuning knob, and if so, what will it do and how will it be used.
Those that insist there is no cause to have any such device are apt to equally insist that we must have AI that is only and always practicing the utmost of ethical behavior.
Is that a Utopian perspective or can it be achieved in the real world as we know it?
Only my crystal ball can say for sure.
Copyright 2020 Dr. Lance Eliot
This content is originally posted on AI Trends.
[Ed. Note: For reader’s interested in Dr. Eliot’s ongoing business analyses about the advent of self-driving cars, see his online Forbes column: https://forbes.com/sites/lanceeliot/]
http://ai-selfdriving-cars.libsyn.com/website
from AI Trends https://ift.tt/37zf9mw
via A.I .Kung Fu