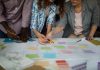
By Dawn Fitzgerald, the AI Executive Leadership Insider
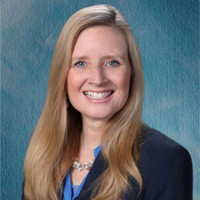
Part Three of Four Part Series: “AI Holistic Adoption for Manufacturing and Operations” is a four-part series which focuses on the executive leadership perspective including key execution topics required for the enterprise digital transformation journey and AI Holistic Adoption for manufacturing and operations organizations. Planned topics include: Value, Program, Data and Ethics. Here we address our third topic: Data.
The Executive Leadership Perspective
For the executive leader who is taking their enterprise on a journey of Digital Transformation and AI Holistic Adoption, we started this series with the foundation of Value and then moved to the framework of the Program. Although these are the fundamental building blocks required for success, the results of any enterprise’s analytics, do, in the end, rely on the Data.
The executive leader has the responsibility to ensure that they and their team are dedicated to mastering data fluency and data excellence in the enterprise. The facets of Data Management are vast with the standard areas of focus including data discovery, collection, preparation, categorization and protection. Strategies for achieving maturity in these areas are well-established in most industries, and yet many industries still struggle. These standard areas of focus in Data Management are indeed necessary but are not sufficient for the needed AI Holistic Adoption.
To incorporate AI Holistic Adoption, a value focus must be employed where we create Value Analytics (VAs) as output from our enterprise Analytics Program. To support this program, we must expand our enterprise Data Management definition to include a Data Optimality metric, a Data Evolution Roadmap and a Data Value Efficiency metric.
The Data Optimality metric tells us how close the Value Analytics (VA) Baseline Dataset is to ‘optimal’. The Data Evolution Roadmap captures the milestones for the evolution of our Baseline Dataset for each Value Analytics release and the corresponding goals for harvesting data. The Data Value Efficiency metric simply measures how much value we achieve from harvested data. The combination of these is a powerful tool set for the executive leader to ensure the data provides the highest value to enterprise analytics at the lowest cost to the organization.
The Data Optimality Metric Definition
The Data Optimality metric tells us how close the Value Analytics (VA) Baseline Dataset is to the Data Scientist-defined ‘optimal’. The Baseline Dataset is a key component to any Value Analytic. The Baseline Dataset captures the data used for the VA as it relates to a specific development release. This link to a release is a critical distinction. By tying the Baseline Dataset to the VA design release, we recognize a snapshot of the training data associated with a specific release. We recognize that it may not be optimal so may change during the lifetime of the VA, and we plan for its change on a Data Evolution Roadmap.
To achieve enterprise AI Holistic Adoption the executive leader must ensure the foundation of Value which anchors the effort. They must also incorporate the nature of a technical development effort. Specifically, they must account for the go-to-market demands that drive risk management decisions regarding minimal viable product (MVP) in Agile or SAFe (Scaled Agile Framework) methodologies. By the very nature of development, the MVP-driven organization will plan early deliverables with incremental improvements over time. This will apply to the Baseline Dataset as well and thus, the Data Optimality Metric is created. It is used for visibility of the state of our Baseline Dataset, used to communicate expectations of its impact on the VA and used to drive the evolution of the data.
Data Optimality Metric Example
To illustrate the power of the Data Optimality metric, consider the Data Scientist who has defined an equipment predictive maintenance algorithm and has a corresponding Baseline Dataset definition. They will have defined the optimal dataset that they want which includes the IoT measurements (for example: temp, pressure and vibration), the duration of time they would like the Data collected over (for example: 6 months), the population size (for example: data collected from 10 Data Centers covering four key climate zone geographies) and a guaranteed data quality level (for example less than 10% data gaps). Since there is a low probability of this optimal Baseline Dataset availability aligning with the market-driven release timeline demands, the Data Scientist may be forced to compromise their initial Baseline Dataset by taking fewer IoT parameters (for example: only temp and pressure but no vibration), having shorter collection duration (for example: 3 months vs 6), having a smaller population size (for example: only 3 Data Centers vs 10) or accepting a lower quality level guarantee. The Data Scientist may also create simulated data for some or all of the data gaps.
The Data Scientist will then assign a Data Optimality metric to the current release Baseline Dataset (for example: current available data achieves 60% of the optimal dataset criteria). They will also state the lower Data Optimality metrics potential impact on the Value Analytic (for example: customers can expect only a 30-day prediction vs 90-day prediction pre-failure).
The executive leader can then make a business decision to go forward with this Data Optimality metric or wait the extra time necessary to harvest improved data to achieve a higher Data Optimality metric and corresponding VA improvement. To conclude this scenario example, input from the marketing team may indicate that a Q2 release of the VA with the current Data Optimality metric is acceptable due to first mover advantage and significant value, compared to competitive offers, delivered to the customer.
They may also specify that the higher Data Optimality metric must be achieved by Q4 in order to remain competitive. The Data Optimality metric enables defined incremental improvements to the Baseline Dataset over time which transcend to the ongoing VA improvement lifecycle.
The visibility provided by the Data Optimality metric is especially valuable with leading edge Value Analytic capabilities where first mover advantage in the market can lead to a substantial market penetration foothold for the business. The metric drives cost saving by bringing the decision point of release impacting information down to the local business, where the knowledge of the business is the highest. This simultaneously gives visibility to future data management actions through the enterprise and should be captured in the Data Evolution Roadmap.
The Data Evolution Roadmap
Driven by Data Optimality metric inputs, the Data Evolution Roadmap captures the milestones for the evolution of our Baseline Dataset for each Value Analytics release and the corresponding goals for harvesting future required data. The Data Evolution Roadmap establishes an enterprise framework that provides visibility, alignment, clarity and flexibility for local business decisions. It also challenges the business to define the Data Optimality metric and track Baseline Dataset improvements.
The power of the Data Evolution Roadmap enables the local businesses’ Agile development methodologies, gives cross-functional visibility of data management actions and delivers Data Management cost saving to the enterprise. Incremental improvements of the Data Optimality metric for a specific Value Analytic can be timed on the Data Evolution Roadmap based on demand. Early market traction data can be incorporated to update the business decision thus generating higher confidence in the data management expenditures and potential cost savings if deemed no longer necessary.
To achieve AI Holistic Adoption, the Data Evolution Roadmap must align directly to the Value Analytics Roadmap. Data management tasks must align and be traceable through both roadmaps to a higher end value. Successful execution of this requires rapid, tightly coupled agile development teams that span the key enterprise stakeholders such as IoT development, Data management, Data Science, platform development and marketing/sales functions. This demand-pull approach to Data Management aligns well with Agile development practices and combats the seemingly overwhelming challenges of exponential data repository growth and corresponding data management costs.
Data Repository Growth
The growth of the data repository should parallel the growth and maturity of the Analytics Program to ensure data excellence and avoid dark data obsolescence. The cost of technical debt must be acknowledged and measured.
Many companies make the mistake of a volume goal of collecting IoT data without a defined data evolution strategy aligned with the Analytics Program grounded in value. This leads to the data swamp, a stalling of the realization of Value from the AI solutions and an overall low Data Value Efficiency score as defined below.
A tighter alignment of the Data Management tasks with the Value Analytics also provides opportunity for more value-based incremental improvements of the enterprises’ tagging strategy. Tagging data with both technical and business metadata is critical but seldom done correctly first pass and certainly not without a Value focus, which requires a cross-functional team of a data architect, data scientist, subject-matter expert and marketing that anchor the value. The mechanism to continuously improve your data tagging methodology must be close to the value goals of the Analytics Program.
The Data Value Efficiency
Once the Data Optimality metric and Data Evolution Roadmap are established, a Digital Value Efficiency (DVE) metric can be measured. The Data Value Efficiency (DVE), a measurement attached to data elements, is simply the measure of how much value we achieve from harvested data. The DVE tracks the use of the data by its inclusion in different VA Baseline Datasets over time.
In most industries using AI, this metric would be considered very low. IDC research defines that currently, “80% of time is spent on data discovery, preparation, and protection, and only 20% of time is spent on actual analytics and getting to insight.” To achieve high DVE, a larger portion of our data harvested must translate into higher value actionable insights.
Since the executive leader’s responsibility is to ensure that the organization is efficient with the data management, they must focus their organization on shifting the percentage of time invested from data discovery, collection and preparation to a higher amount of time used in training models and insight generation. The DVE metric gives visibility to progress toward this goal.
The Data Evolution Roadmap pivots the enterprise focus from one of maximum data collection, and corresponding cost, to one of minimized data collection driven by the Value Analytics roadmap. Over time, this will improve the DVE metric and overall data excellence of the enterprise.
Dawn Fitzgerald is VP of Engineering and Technical Operations at Homesite, an American Family Insurance company, where she is focused on Digital Transformation. Prior to this role, Dawn was a Digital Transformation & Analytics executive at Schneider Electric for 11 years. She is also currently the Chair of the Advisory Board for MIT’s Machine Intelligence for Manufacturing and Operations program. All opinions in this article are solely her own and are not reflective of any organization.
from AI Trends https://ift.tt/38CCnZb
via A.I .Kung Fu
No comments:
Post a Comment